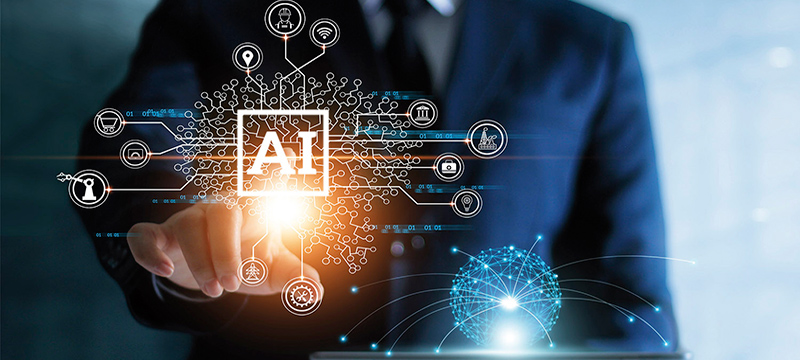
Retrieval Augmented Generation: The Secret to Building Smarter, More Adaptive AI Systems
Retrieval Augmented Generation (RAG) is an innovative approach in artificial intelligence that combines the strengths of retrieval-based systems with generative models to create more responsive and intelligent AI systems. By integrating these two capabilities, RAG technology enhances the ability of AI to provide accurate and contextually relevant responses, making it a pivotal advancement in modern AI development.
RAG stands out from traditional language models by addressing their limitations in static knowledge representation. Unlike conventional models that rely solely on pre-trained data, RAG models dynamically retrieve and incorporate external information, allowing for real-time context enhancement. This capability makes RAG an essential development in creating AI systems that are not only smarter but also more adaptive to new information and contexts.
The Core Mechanism of RAG
At the heart of RAG is its ability to gather and integrate information dynamically. This retrieval-based information gathering involves accessing external databases to fetch relevant data based on the context of a query. By doing so, RAG models can enhance their responses with up-to-date and precise information, improving overall accuracy and relevance.
Moreover, RAG’s dynamic knowledge integration allows it to seamlessly blend new data with existing model outputs. This process ensures that AI systems can adapt to changes and deliver responses that are contextually appropriate. For a deeper understanding of how this mechanism works, you can explore what is retrieval augmented generation.
Evolution of AI Language Models
Traditional AI language models have been limited by their static nature, relying on a fixed dataset for generating responses. This limitation necessitated the development of adaptive knowledge systems capable of integrating external information in real-time. RAG emerges as a breakthrough solution, offering a way to overcome these constraints and enable AI systems to evolve alongside their informational environments.
Technical Architecture of RAG Systems
The technical architecture of RAG systems is composed of several critical components that work together to facilitate efficient information retrieval and response generation. This architecture includes sophisticated mechanisms for retrieving data and integrating external knowledge bases, ensuring that AI systems can adapt and respond accurately to complex queries.
Retrieval Mechanisms
RAG systems utilize advanced vector database technologies to store and retrieve information efficiently. These technologies enable the system to perform semantic searches, which are crucial for understanding the context and nuances of a query. By employing relevance ranking algorithms, RAG systems can prioritize and select the most pertinent information from a vast pool of data.
Generation and Response Synthesis
Once the relevant information is retrieved, RAG systems focus on generating contextual responses. This process involves synthesizing data to produce coherent and accurate outputs. Enhancements in accuracy and coherence are achieved by leveraging dynamic content integration, allowing RAG systems to handle even the most complex query scenarios effectively.
Practical Applications and Future Potential
RAG technology holds significant promise across various industries, offering innovative solutions to long-standing challenges. By facilitating real-time data integration and adaptive response generation, RAG systems are poised to revolutionize numerous sectors.
Enterprise AI Solutions
In the realm of enterprise AI, RAG systems are being utilized to automate customer support processes, manage extensive knowledge bases, and personalize information retrieval. These applications not only enhance operational efficiency but also improve user experience by delivering timely and relevant information.
Emerging Research and Development
Ongoing research in RAG technology focuses on addressing existing challenges and exploring new frontiers. Researchers are working to overcome obstacles such as data privacy concerns, computational efficiency, and the need for more nuanced understanding in AI systems. As innovations continue, RAG is expected to play a transformative role in the future, driving AI advancements that align with evolving technological and societal needs.
Retrieval Augmented Generation represents a significant leap forward in the development of AI language models. By enabling systems to adapt dynamically and incorporate real-time information, RAG technology is paving the way for smarter, more responsive AI solutions that are well-equipped to meet the demands of an ever-changing digital landscape.
The post Retrieval Augmented Generation: The Secret to Building Smarter, More Adaptive AI Systems appeared first on IoT Business News.